Deepfakes are changing the way we see videos and images – we can no longer trust photographs or video footage. Advanced AI methods now enable the generation of highly realistic images, making it increasingly difficult, or even impossible, to distinguish between what’s real and what’s digitally created.
In the following, we will explain what Deepfakes are, how to identify them, and discuss the impact of AI-generated photos and videos.
What are Deepfakes?
Deepfakes represent a form of synthetic media wherein an individual within an existing image or video is replaced or manipulated using advanced artificial intelligence techniques. The primary objective is to create alterations or fabrications that are virtually indistinguishable from authentic content.
This sophisticated technology involves the use of deep learning algorithms, specifically generative adversarial networks (GANs), to blend and manipulate visual and auditory elements, resulting in highly convincing and often deceptive multimedia content.
Technical advances and the release of powerful tools for creating deepfakes are impacting various domains. This raises concerns about misinformation and privacy, along with the need for strong detection and authentication mechanisms.

History and Rise of Deep-Fake Technology
The concept emerged from academic research in the early 2010s, focusing on facial recognition and computer vision. In 2014, the introduction of Generative Adversarial Networks (GANs) marked a major advancement in the field. This breakthrough in deep learning technologies enabled more sophisticated and realistic image manipulation.
Advancements in AI algorithms and machine learning are fueling the rapid evolution of deepfakes. Not to mention the increasing availability of data and computational power. Early deepfakes required significant skill and computing resources. This means that only a small group of highly specialized individuals were capable of creating them.
However, this technology is becoming increasingly accessible, with user-friendly deepfake creation tools enabling wider usage. This democratization of deepfake technology has led to an explosion in both creative and malicious uses. Today, it’s a topic of significant public interest and concern, especially in the face of political election cycles.
The Role of AI and Machine Learning
AI and machine learning, particularly GANs, are the primary technologies for creating deepfakes. These networks involve two models: a generator and a discriminator. The generator creates images or videos while the discriminator evaluates their authenticity.
Through iterative training, the generator continuously improves its output to fool the discriminator. This continues until the system eventually produces highly realistic and convincing deepfakes.
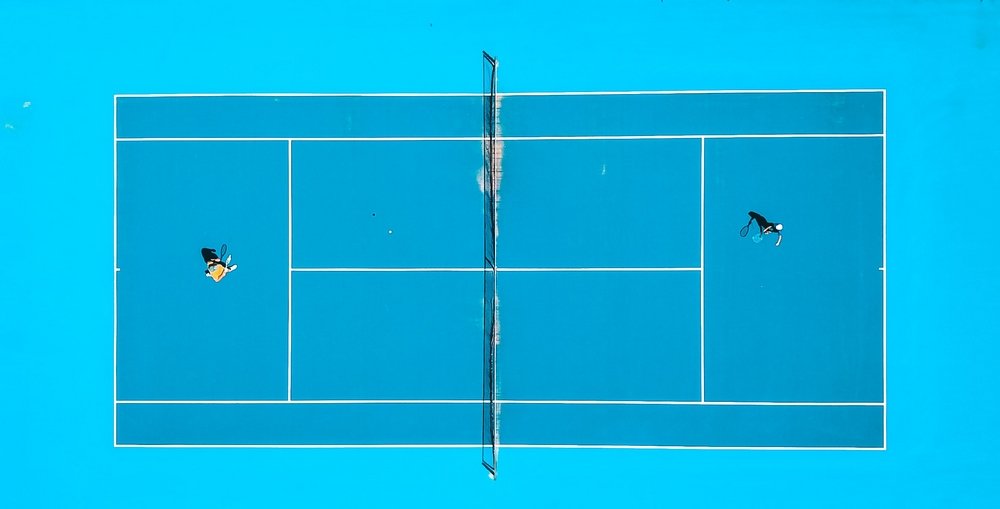
Another crucial aspect is the training data. To create a convincing deepfake, the AI requires a massive dataset of images or videos of the target individual. The quality and variety of this data significantly influence the realism of the output.
AI models and deep learning algorithms analyze this data, learning intricate details about a person’s facial features, expressions, and movements. The AI then attempts to replicate these aspects in other contexts or onto another person’s face.
While it holds immense potential in areas like entertainment, journalism, and social media, it also poses significant ethical, security, and privacy challenges. Understanding its workings and implications is crucial in today’s digital age.
Deepfakes in the Real World
Deepfakes are becoming harder to distinguish from reality while simultaneously becoming more commonplace. This is leading to friction between proponents of its potential and those with fundamental ethical concerns about its use.
Entertainment and Media: Changing Narratives
Deepfakes are opening new avenues for creativity and storytelling. Filmmakers or content creators can use the likeness of individuals or characters without their actual presence.
AI offers the ability to insert actors into scenes post-production or resurrect deceased celebrities for new roles. However, it also raises ethical questions about consent and artistic integrity. It also blurs the line between reality and fiction, potentially misleading viewers.
Filmmakers are using deep fake technology in mainstream content, including blockbuster films:
- Martin Scorsese’s “The Irishman”: An example of using deepfake technology to de-age actors.
- Star Wars: Rogue One: AI was used to produce the deceased actor Peter Cushing’s character, Grand Moff Wilhuff Tarkin.
- Roadrunner: In this Anthony Bourdain biopic, audio deepfake tech (voice cloning) was used to synthesize his voice.
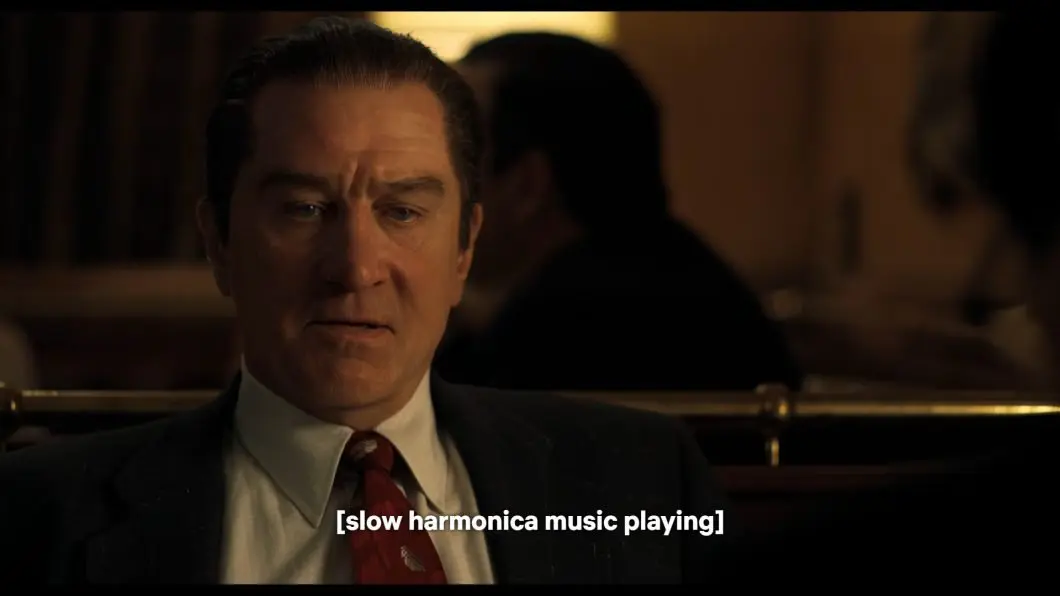
The increasing use of AI generation led to the SAG-AFTRA (Screen Actors Guild‐American Federation of Television and Radio Artists) strike. Actors raised concerns regarding losing control over their digital likenesses and being replaced with AI-generated performances.
Political and Cybersecurity: Influencing Perceptions
Deepfakes in the political arena are a potent tool for misinformation. It’s capable of distorting public opinion and undermining democratic processes en masse. It can create alarmingly realistic videos of leaders or public figures, leading to false narratives and societal discord.
A well-known example of political deepfake misuse was the altered video of Nancy Pelosi. It involved slowing down a real-life video clip of her, making her seem impaired. There are also instances of audio deepfakes, like the fraudulent use of a CEO’s voice, in major corporate heists.
One of the most notable instances of an AI-created political advertisement in the United States occurred when the Republican National Committee released a 30-second ad that was disclosed as being entirely generated by AI.
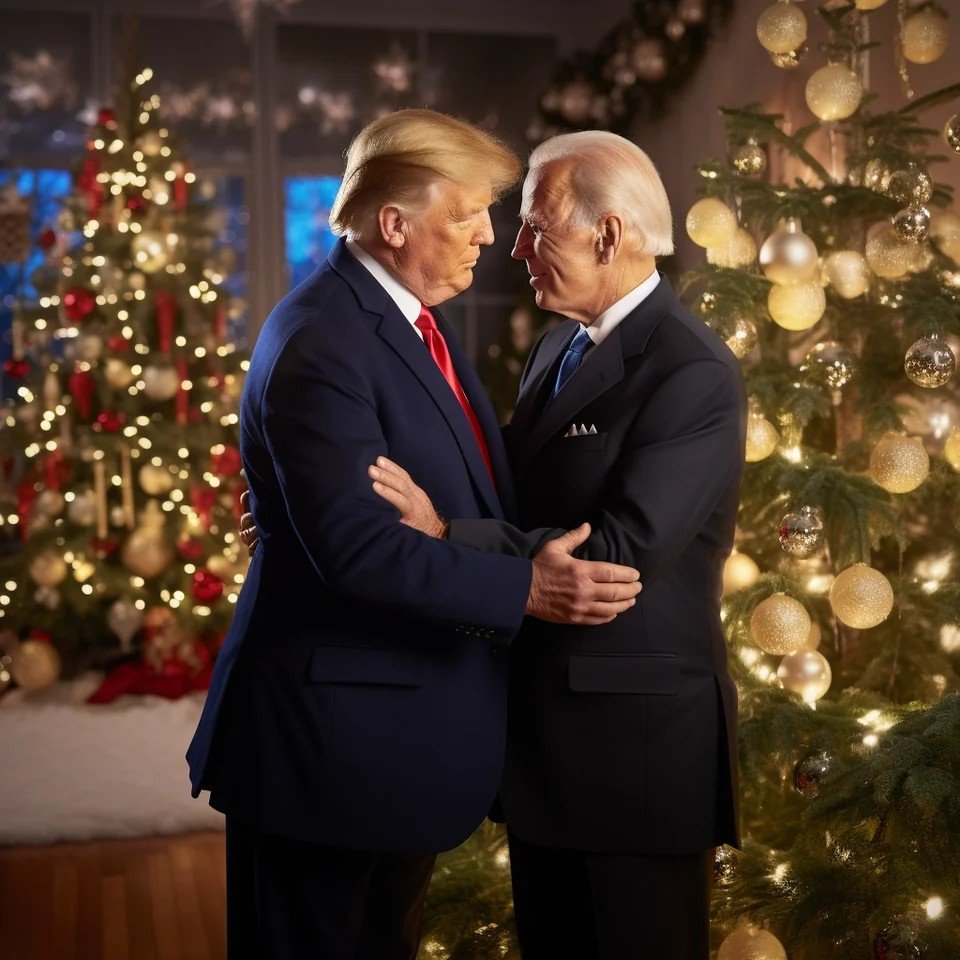
Business and Marketing: Emerging Uses
In the business and marketing world, deepfakes offer a novel way to engage customers and tailor content. But, they also pose significant risks to the authenticity of brand messaging. Misuse of this technology can lead to fake endorsements or misleading corporate communications. This has the potential to subvert consumer trust and harm brand reputation.
Marketing campaigns are utilizing deep fakes for more personalized and impactful advertising. For example, audio deepfakes further extend these capabilities, enabling the creation of synthetic celebrity voices for targeted advertising. An example is David Beckham’s multilingual public service announcement using deepfake technology.
The Dark Side of Deepfakes: Security and Privacy Concerns
The impact of deepfake technology on our society is not limited to our media. It has the potential as a tool of cybercrime and to sow public discord on a large scale.
Threats to National Security and Individual Identity
Deepfakes pose a significant threat to individual identity and national security.
From a national security perspective, deepfakes are a potential weapon in cyber warfare. Bad actors are already using them to create fake videos or audio recordings for malicious purposes like blackmail, espionage, and spreading disinformation. By doing so, actors can weaponize deepfakes to create false narratives, stir political unrest, or incite conflict between nations.
It’s not hard to imagine organized campaigns using deepfake videos to sow public discord on social media. Artificially created footage has the potential to influence elections and heighten geopolitical tensions.
Personal Risks and Defamation Potential
For individuals, the broad accessibility to generative AI leads to a heightened risk of identity theft and personal defamation. One recent case highlights the potential impact of manipulated content: A Pennsylvania mother used deepfake videos to harass members of her daughter’s cheerleading team. The videos caused significant personal and reputational harm to the victims. In consequence, the mother was found guilty of harassment in court.
Impact on Privacy and Public Trust
Deepfakes also severely impact privacy and erode public trust. As the authenticity of digital content becomes increasingly questionable, the credibility of media, political figures, and institutions is undermined. This distrust pervades all aspects of digital life, from fake news to social media platforms.
An example is Chris Ume’s social account, where he posts deepfake videos of himself as Tom Cruise. Although it’s only for entertainment, it serves as a stark reminder of how easy it is to create hyper-realistic deepfakes. Such instances demonstrate the potential for deepfakes to sow widespread distrust in digital content.
The escalating sophistication of deepfakes, coupled with their potential for misuse, presents a critical challenge. It underscores the need for concerted efforts from technology developers, legislators, and the public to counter these risks.
Yes he CANADA! 🐳 @milesfisher @vfxchrisume #TED2023 pic.twitter.com/TyJvhz7a2k
— Metaphysic.ai (@Metaphysic_ai) April 17, 2023
How to Detect Deepfakes
The ability to detect deepfakes has been an important topic in research. An interesting research paper discusses the methods of deepfake recognition in the field of digital face manipulations. The study details various types of facial manipulations, deepfake techniques, public databases for research, and benchmarks for detection tools. In the following, we will highlight the most important findings:
Key Findings and Detection Methods:
- Controlled Scenarios Detection: The study shows that most current face manipulations are easy to detect under controlled scenarios. In other words, when testing deepfake detectors in the same conditions you train them. This results in very low error rates in manipulation detection.
- Generalization Challenges: There’s still a need for further research on the generalization ability of fake detectors against unseen conditions.
- Fusion Techniques: The study suggests that fusion techniques at a feature or score level could provide better adaptation to different scenarios. This includes combining different sources of information like steganalysis, deep learning features, RGB depth, and infrared information.
- Multiple Frames and Additional Information Sources: Detection systems that use face-weighting and consider multiple frames, as well as other sources of information like text, keystrokes, or audio accompanying videos, could significantly improve detection capabilities.
Areas Highlighted for Improvement and Future Trends:
- Face Synthesis: Current manipulations based on GAN architectures, like StyleGAN, provide very realistic images. However, most detectors can distinguish between real and fake images with high accuracy due to specific GAN fingerprints. Removing these fingerprints is still challenging. One challenge is adding noise patterns while maintaining realistic output.
- Identity Swap: It’s difficult to determine the best approach for identity swap detection due to varying factors, such as training for specific databases and levels of compression. The study highlights the poor generalization results of these approaches to unseen conditions. There is also a need to standardize metrics and experimental protocols
- DeepFake Databases: The latest DeepFake databases, like DFDC and Celeb-DF, show high performance degradation in detection. In the case of Celeb-DF, AUC results fall below 60%. This indicates a need for improved detection systems, possibly through large-scale challenges and benchmarks.
- Attribute Manipulation: Similar to face synthesis, most attribute manipulations are based on GAN architectures. There is a scarcity of public databases for research in this area and a lack of standard experimental protocols.
- Expression Swap: Facial expression swap detection is primarily focused on the FaceForensics++ database. The study encourages the creation and publication of more realistic databases using recent techniques.
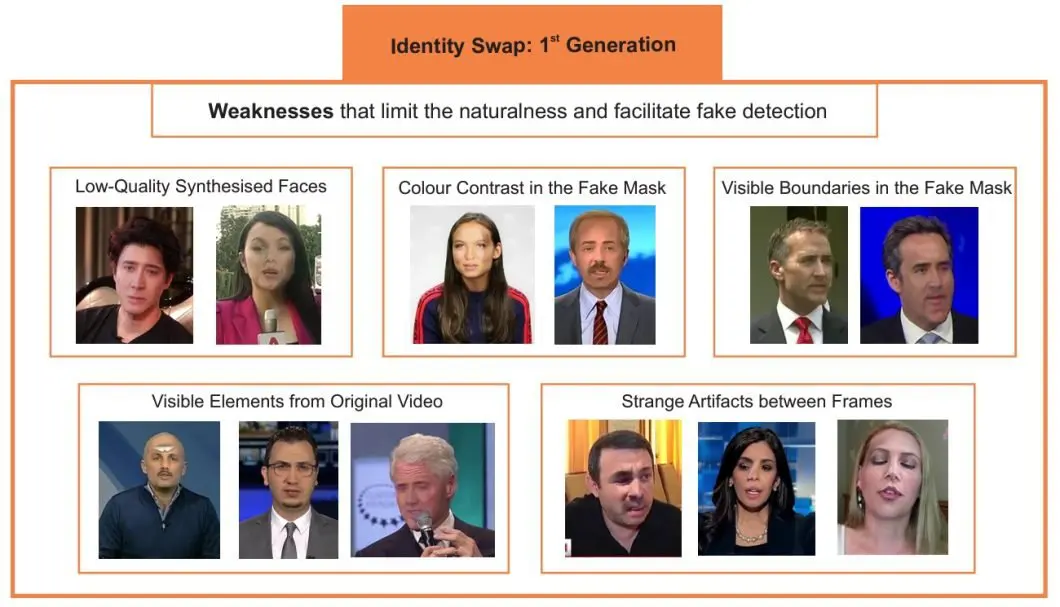
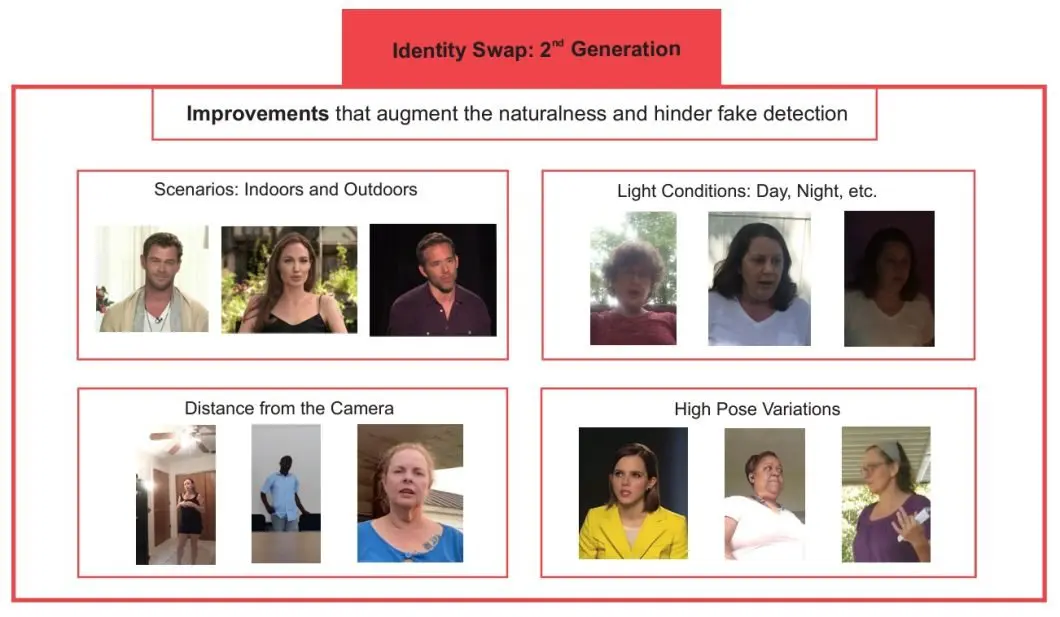
How to Mitigate the Risks of Deepfakes
Despite their potential to open new doors in terms of content creation, there are serious concerns regarding ethics in deepfakes. To mitigate the risks, individuals and organizations must adopt proactive and informed strategies:
- Education and Awareness: Stay informed about the nature and capabilities of deepfake technology. Regular training sessions for employees can help them recognize potential threats.
- Implementing Verification Processes: Verify the source and authenticity before sharing or acting on potentially manipulated media. Use reverse image searching tools and fact-checking websites.
- Investing in AI-Generated Content Detection Technology: Organizations, particularly those in media and communications, should invest in advanced deepfake detection software to scrutinize content. For example, there are commercial and open-source tools that can distinguish between a human voice and AI voice.
- Legal Preparedness: Understand the legal implications and prepare policies to address potential misuse. This includes clear guidelines on intellectual property rights and privacy.
Outlook and Future Challenges for Deepfakes
At a global level, we expect a much broader use of AI generation tools. Even today, a study conducted by Amazon Web Services (AWS) AI Lab researchers found that a “shocking amount of the web” is poor-quality AI-generated content.
collective action is crucial to address associated challenges:
- International Collaboration: Governments, tech companies, and NGOs must collaborate to standardize deepfake detection and establish universal ethical guidelines.
- Developing Robust Legal Frameworks: There’s a need for comprehensive legal frameworks that address deepfake creation and distribution while respecting freedom of expression and innovation.
- Fostering Ethical AI Development: Encourage the ethical development of AI technologies, emphasizing transparency and accountability in AI algorithms used for media.
The future outlook in countering deepfakes hinges on balancing technological advancements with ethical considerations. This is challenging, as innovation tends to outpace our ability to adapt and regulate fields like AI and machine learning. However, it’s vital to ensure innovations serve the greater good without compromising individual rights and societal stability.