Find the best and most valuable applications of computer vision technology for Smart City solutions and Smart Towns in 2024. This article includes novel, practical, and critical real-world use cases with cameras and computer vision technologies.
In this article, we will cover the following
- The basis: What is Computer Vision
- New trends: Deep Learning and Edge AI
- Safety Applications in Smart Cities
- Pandemic control and compliance
- Traffic and infrastructure monitoring
- How to get started
About us: Viso.ai provides the leading end-to-end Computer Vision Platform Viso Suite. Our AI infrastructure is used by cities and industry leaders to build, deploy, operate, and secure their computer vision applications at scale. Get a demo for your organization.
Computer Vision in Smart Cities
In recent years, Artificial Intelligence (AI) for smart cities has emerged with the integration of advanced technologies such as the Internet of Things (IoT), Deep Learning, and Cloud Computing. These technologies offer great potential in addressing the challenges faced by cities in terms of infrastructure and society. With smart technologies, cities can enhance energy distribution, streamline waste management, reduce traffic congestion, improve air quality, and more with the help of smart, connected sensing systems.
Smart city technologies must be highly scalable and connected to function effectively at multiple, dispersed locations. The recent advancements in computer vision, including Edge AI and Deep Learning, combine AI and vision with IoT to create AIoT. These new technologies enable the handling of large amounts of complex visual data, ensuring fast processing, robustness through decentralization, and scalability of computer vision systems in real-world applications.
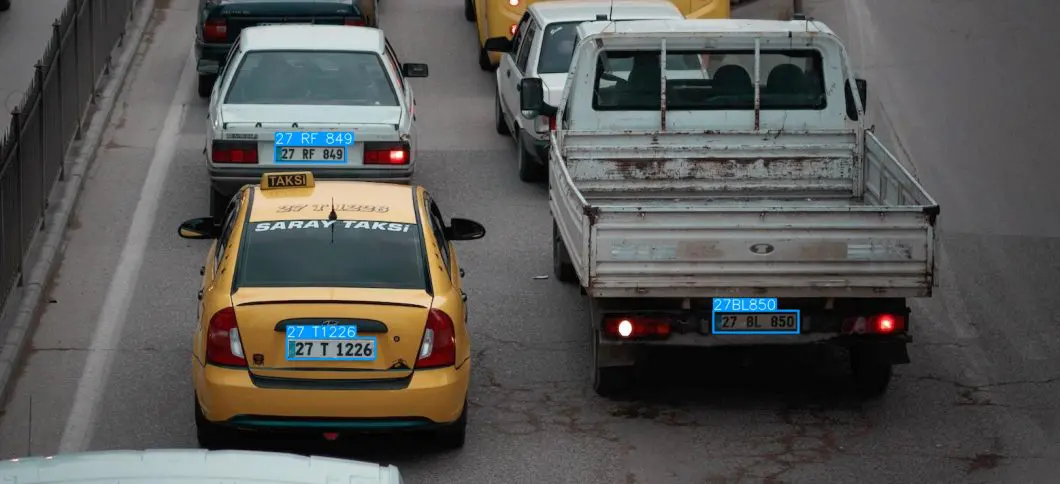
AI, Computer Vision, and Image Recognition
Inside the field of Artificial Intelligence, Computer Vision is a subfield that includes technologies allowing computers to “learn” to recognize a picture or the characteristics of images. Image recognition allows identifying objects, humans, animals, or a position in an image or video feed.
The goal of Computer Vision is that a machine can understand the world and provide information to automate tasks based on this data. New machine learning technologies, most prominently Deep Learning, brought significant breakthroughs to the field of image recognition, making AI vision robust and useful for mission-critical business applications.
Compared to traditional Machine Vision, Deep Learning does not require special imaging cameras and can provide accurate results with almost any digital camera or even webcam. Hence, smart city applications of computer vision often include already installed network cameras (IP cameras or CCTV cameras) to provide the input for real-time video analytics with AI models.
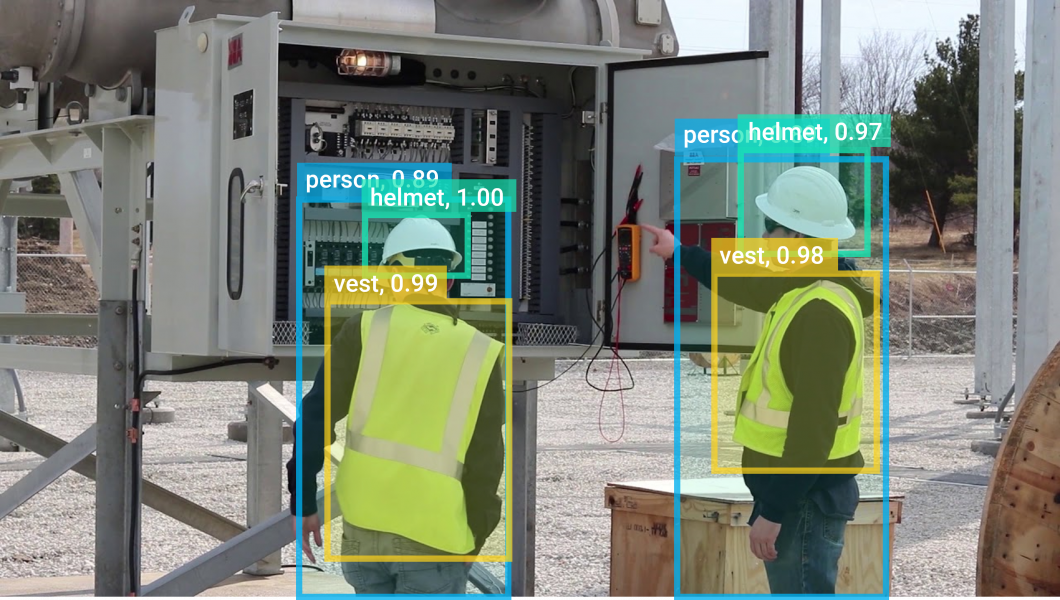
Edge Computer Vision
The latest trend, Edge AI, moves machine learning from the cloud to multiple connected edge devices (physical computers) connected to cameras and processes the data on-device (Edge Intelligence). This approach is based on Edge Computing in connection with the Internet of Things (IoT) devices for remote management.
Edge AI helps to overcome the limitations of the cloud and enables high-performing, robust, real-time, and private computer vision applications – making it possible to use Computer Vision at scale in the real world.
Compared to other sensor technologies such as RFID, GPS, and UWB or BLE that require the installation of sensors to all entities, computer vision is non-invasive, comparably easy to implement and scale, and provides much more information (location, context, semantic information, multi-dimension perspective).
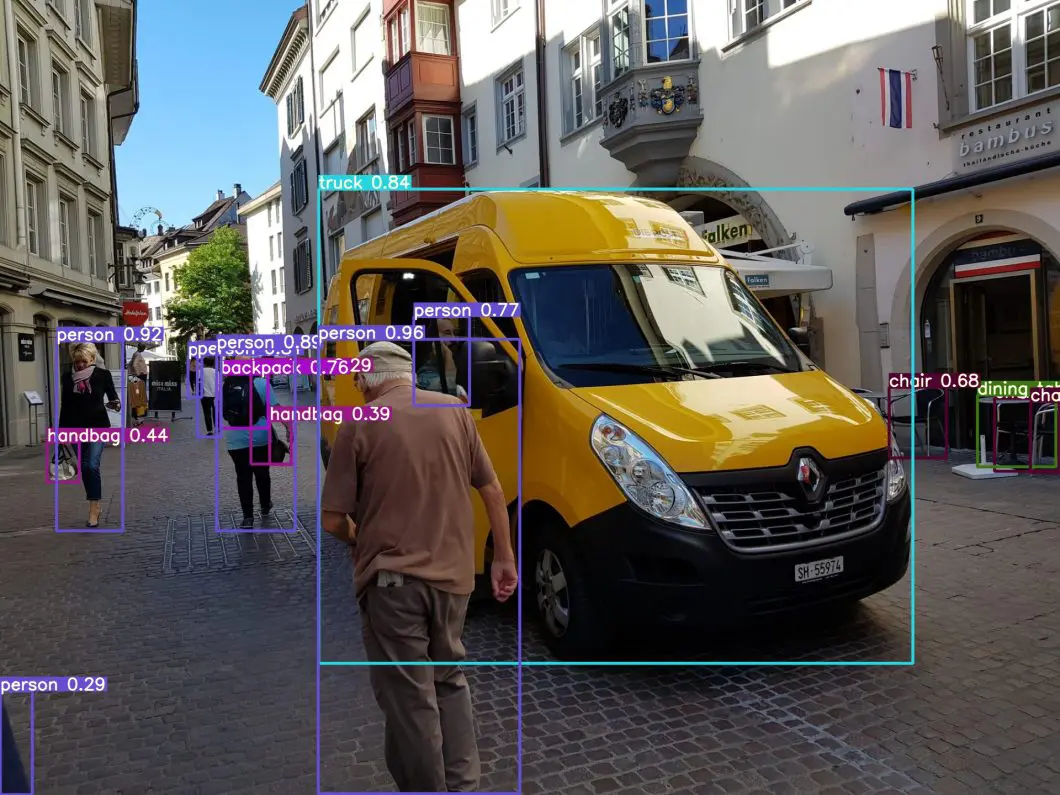
Top Smart City Computer Vision Applications
In the following, I will provide an extensive list of computer vision examples and deep learning use cases in smart cities. The following high-value applications include security and safety, pandemic control strategies, and traffic and infrastructure monitoring tasks.
The full potential of cloud-based IoT applications is largely untapped. Connected smart vision systems can analyze, manage, and collect data in real-time. This aids in helping improve the quality of life, make better decisions, improve sustainability, and cut costs to increase efficiency.
- Application #1: Perimeter monitoring and Person detection
- Application #2: Detect violent and dangerous situations
- Application #3: Action Recognition for Vandalism Detection
- Application #4: Compliance Monitoring and Inspection
- Application #5: Suicide prevention in public spaces
- Application #6: Crowd disaster avoidance application
- Application #7: Protection of Critical Infrastructures
- Application #8: Weapon detection and reporting
- Application #9: Social Distancing monitoring in public places
- Application #10: Automated mask detection
- Application #11: Hygiene Compliance Control
- Application #12: Healthcare Monitoring
- Application #13: Smart city traffic monitoring
- Application #14: Traffic rule violation detection
- Application #15: Roadside surveillance application
- Application #16: Parking Lot Occupancy Detection
To find related applications, check out the report about computer vision in energy and utility applications.
Safety and Security Applications for Smart Cities
1. Perimeter Monitoring and Person Detection
The detection of people has a wide range of applications in smart cities. Applications include computer vision methods for real-time video analysis to interpret human activity. Other computer vision applications are used to detect people and activity recognition in restricted areas at airports or rail stations.
Deep Learning systems are used to detect intrusion events in real-time through the perimeter and identify the target’s position. Such automated, AI-based perimeter monitoring systems can efficiently cover large monitoring areas for safety and security applications.
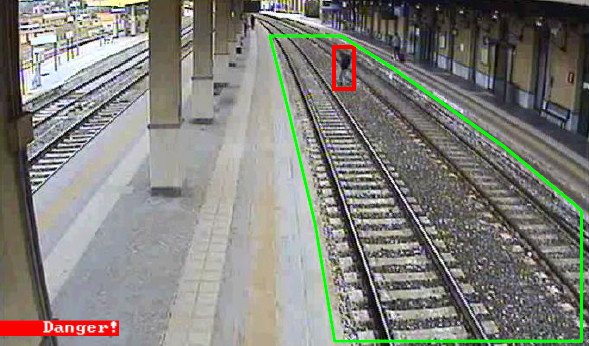
Detect intrusion events in pre-defined areas by identifying the target’s position, date and time.
2. Detect Violent and Dangerous Situations
Computer Vision in smart city applications often involves the automatic detection of dangerous situations to ensure the safety of residents with smart video surveillance. Such dangerous situations can be fighting, brawls, robberies, and more. The strong variability of such scenes is a challenging problem.
Algorithms are used for detecting people, tracking people, and estimating three-dimensional human poses (Human Pose Estimation), all to recognize the actions and interactions of people. The output of AI models is processed with a logic flow to define scenarios and events that should trigger human intervention or provide analytics. Such applications involve people counting and the detection of people who spend an unusual amount of time in specific urban areas (dwell time analysis).
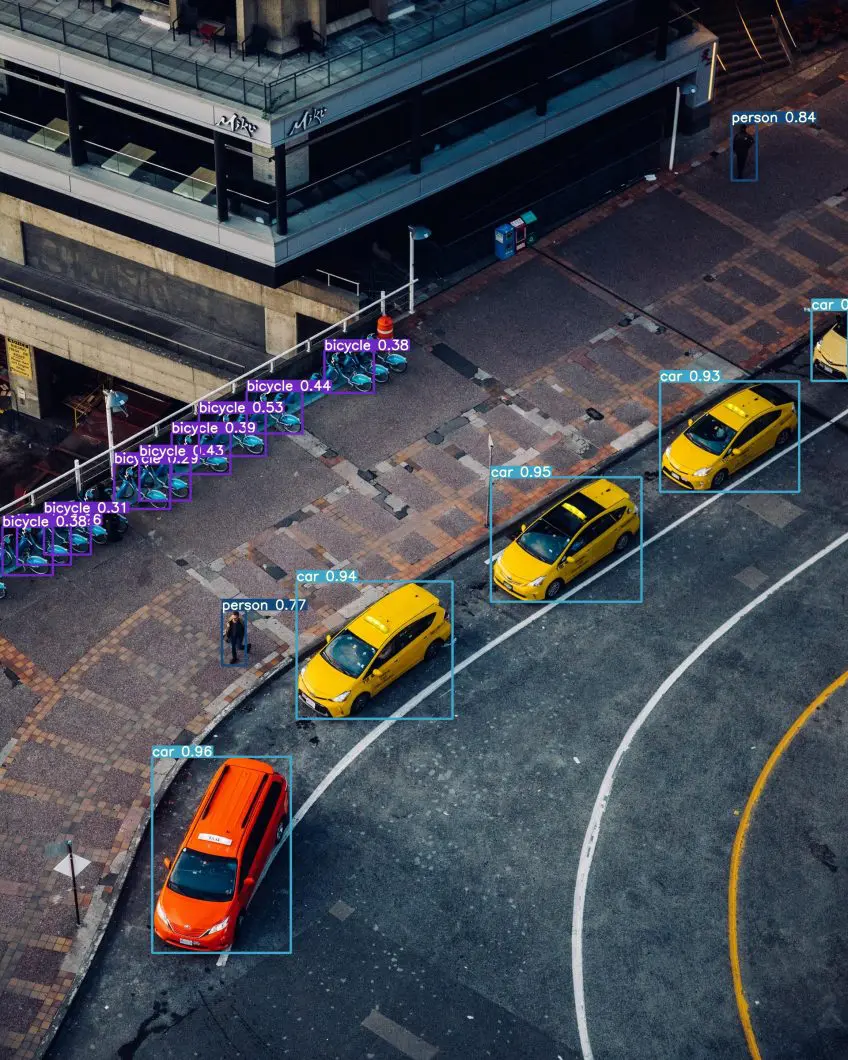
3. Action Recognition for Vandalism Detection
Different machine learning methods can be used in action recognition applications. For example, machine learning and feature extraction techniques have been introduced for human behavior monitoring and support. Vision-based technologies can recognize human behaviors such as fighting and vandalism events that may occur in a public district using one or numerous camera views. Computer vision systems can be used to detect and predict suspicious and aggressive behaviors in real time, even in complex and crowded environments.
There are increasing concerns about trends in vandalism and interference with ancient sites and heritage buildings. The preservation of historic sites and their restorations is of great importance to communities and a significant factor for tourism. Hence, intelligent vision systems can help to protect heritage buildings and sites.
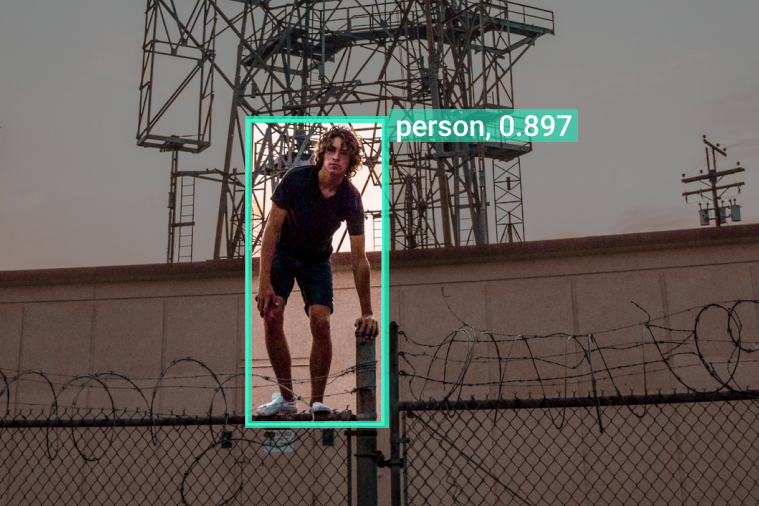
4. Safety and compliance Control and Inspection with Deep Learning
There is a wide range of compliance monitoring use cases with computer vision in a smart city. Since manual monitoring of compliant and unsafe conditions is very challenging, labor-intensive, and expensive, AI vision methods offer automated and scalable alternatives to manual inspection and site observations.
Furthermore, computer vision methods are not only more time-efficient but also more accurate. Expert safety officers are usually not always present and make inspection processes unreliable for regular practice. Computer vision algorithms can be used to perform continuous monitoring of complex, large-scale sites.
In construction jobs, the workforce faces the highest probability of injury or death on job sites, 5x more compared to other industries. Numerous injuries can be avoided if workers always wear proper Personal Protective Equipment (PPE) during work, including helmets, safety glasses, vests, hand gloves, steel toe boots, etc. To minimize accidents, compliance monitoring is essentially required to detect situations of workers not wearing proper PPE or constant norm violations effectively.
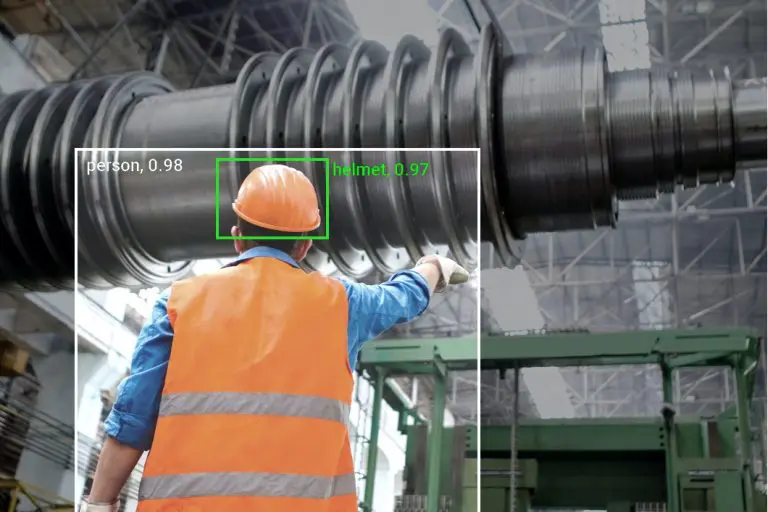
Automated safety and compliance monitoring to minimize accidents and increase efficiency.
5. Suicide Prevention in Public Spaces
Camera systems are used to automate suicide prevention in public spaces by classifying visual features, characterizing body joint movements, and identifying unusual behavior. CCTV cameras with deep learning smart city applications can be used for assessing crisis behaviors at hotspots such as metro stations. Therefore, automated computer vision applications can help to identify self-harm attempts in progress and initiate an intervention.
The main goal is the development of automated detection systems for early intervention and detection of pre-attempt behaviors. Therefore, computer vision applications can help to identify risk factors, for example, by inferring depression from facial expressions using facial analytics. Another way is to detect unusual behavior and movement patterns or waiting times at specific hotspots. Also, forensic vision applications are used to understand factors after an attempt.
6. Crowd Disaster Avoidance Application
Computer vision surveillance in crowded scenes is one of the most important applications due to the increase of people gatherings in public places where the possibilities of disasters and stampedes are also high. Therefore, vision-based crowd disaster avoidance systems are used to improve public safety.
In general, such applications focus on crowd scene analysis and behavior analysis. Stampedes may occur due to the abnormal behavior of individuals or unexpected events. Deep learning models are used to count people, and estimate crowd density with one or multiple cameras at scale.

Area-based people counting in real-time using common surveillance cameras.
7. Protection of Critical Infrastructures in Smart Cities
Smart cities use intelligent computing technologies to monitor activities or prevent potential risks. Critical infrastructures are essential resources for society, and their failure would have a very high impact and cost. These include roads, communications, water, energy, and more.
While closed-circuit television (CCTV) systems have become an essential element for security and law enforcement, traditional surveillance systems depend on the attention capacity of a human operator who is confronted with a multitude of video streams.
The main limitations of conventional video surveillance systems include high operating costs due to the need for personnel to watch the videos, fatigue-related errors, and an enormous amount of video data demanding high bandwidths. Thus, new Edge AI technologies enable distributed sensing systems that achieve real-time performance, cost-efficiency, and scalability required to implement large-scale systems.
Edge AI computer vision makes it possible to run machine learning on-device with local edge nodes that communicate to the Cloud (AIoT). Such edge computers can be any computer or embedded system capable of running high-performance video processing in real time. There is a wide range of edge devices suitable for edge computer vision (e.g., Nvidia Jetson TX2).
In cities, automatic video analysis leverages deep learning in applications such as perimeter monitoring, loitering detection, or multi-person tracking with re-identification.
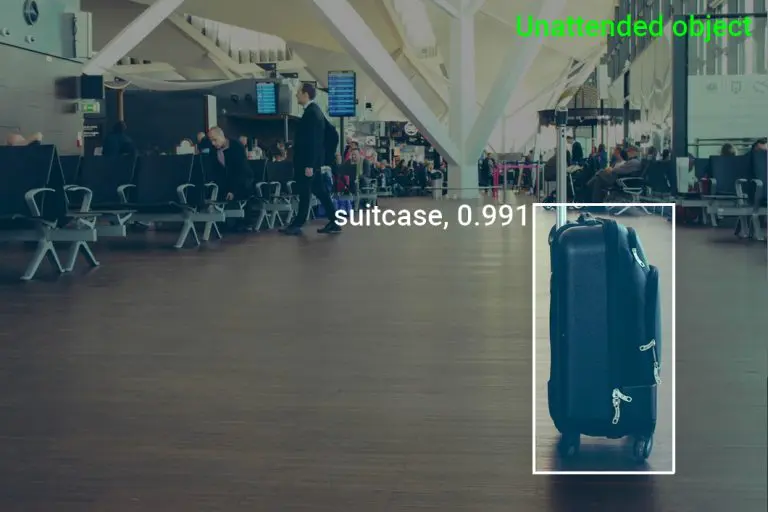
Automatically identify suspicious or dangerous objects placed in public places.
8. AI-based Automated Weapon Detection and Reporting
Mega-cities with dense urban populations face huge challenges in controlling the increasing crime rate. Therefore, deep-learning vision applications can be developed for autonomous surveillance in public places to detect handheld arms in real time. AI models analyze video streams to perform object detection with all objects visible in the camera streams. The weapon detection application triggers an alert when any type of weapon is visible and can track the movement of the object.
This approach can be used to develop an automated firearm detection system. High-performance systems are capable of detecting and tracking the person holding the weapon and using the information to perform facial recognition.
A related smart city computer vision use case involves the automated detection of suspicious objects that have been placed in public places. AI models, such as the popular Convolutional Neural Network model YOLOv3 and the recent YOLOR, can be used to detect placed objects that could pose a thread and flag it for human review.
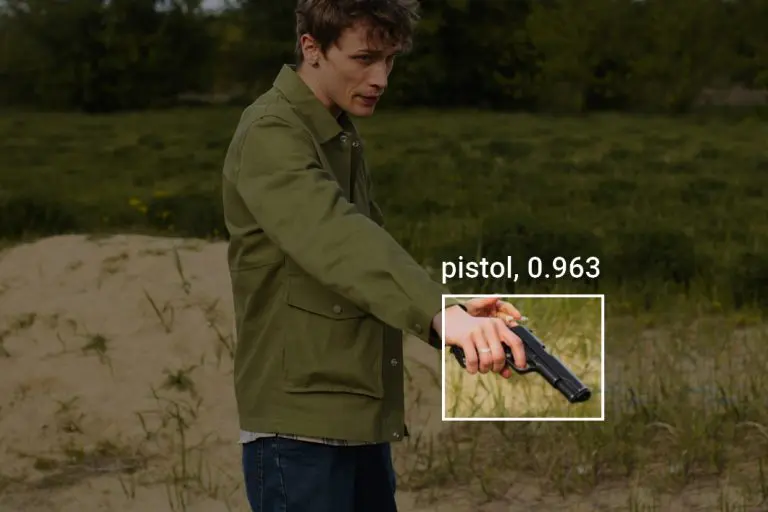
Automatically detect weapons in real-time video streams.
COVID-19 Pandemic Measures for a Smart City
The coronavirus pandemic has revealed the limitations of existing city structures. Hence new systems and technologies such as computer vision provide fast and effective mechanisms to limit the impact of pandemic threats.
The investment in smart city initiatives can enhance the planning and preparation ability to combat pandemics now and in the future. Technological advances offer alternative methods to physical connectivity to maintain and improve urban functionality.
9. Social Distancing Monitoring in Public Places
Smart vision systems are capable of monitoring and enforcing social distancing between people to slow the pandemic spread effectively. For example, deep learning based AI models can be used to create applications for social distancing monitoring with real-time object detection to detect people in videos of surveillance cameras at scale.
In crowd detection applications, a deep neural network (DNN) model can automatically detect people in urban public spaces using CCTV security cameras. The people’s movement trajectories are used to identify potentially high-risk areas for planners to redesign the structure of open and public spaces to make them more pandemic-proof.
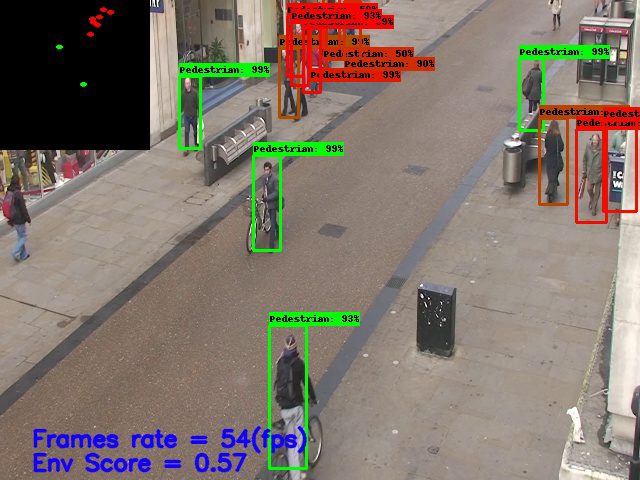
Monitor social distancing between people, identify high-risk areas and non-compliance.
10. Automated Mask Detection
Another application example is automated mask detection of people in public places. AI-enabled systems can facilitate mass surveillance using deep learning algorithms to monitor the conditions and send alerts on certain situations. For example, if people do not wear a mask or do not comply with lock-down measures, in combination with compliance with social distancing protocols in public spaces such as metro stations.
The insights can be used to enhance the capacity of cities to predict pandemic patterns, facilitate a timely response, minimize the transmission of the virus, provide support to specific sectors, minimize supply chain disruption, and ensure the continuation of essential services and operations.
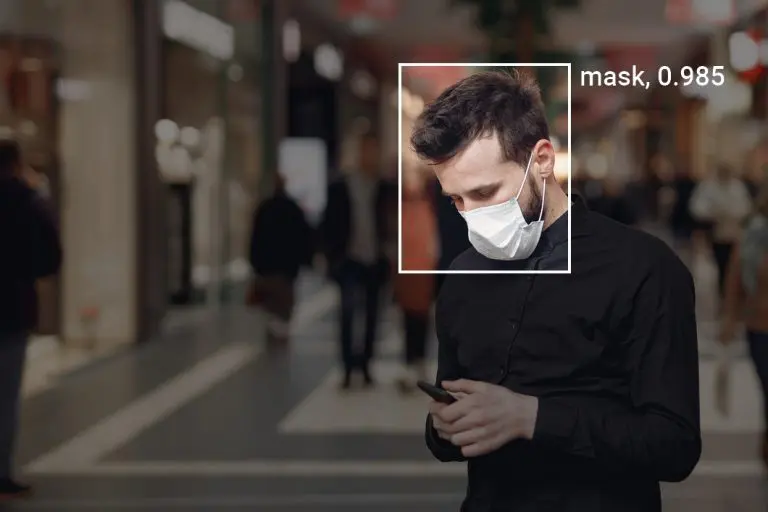
Automatically detect unmasked people in public spaces or indoors.
11. Hygiene Compliance Control with Deep Learning
Computer Vision and Deep Learning based approaches are valuable to automate safety and compliance monitoring. For example, such applications power non-intrusive vision-based systems for tracking people’s activity in public places and infrastructure. AI vision methods have shown better results compared to proximity-based techniques.
An example application is automated hand hygiene compliance monitoring with deep learning. This system conducts spatial analytics to provide insights into human movement patterns. There are promising use cases for reducing hospital-acquired infections.
12. Healthcare Monitoring to Minimize Physical Contact
Face-recognition technologies are used to minimize the need for touching surfaces and objects or physical contact between individuals to reduce the transmission risk. Smart technologies help to reduce the risk of human exposure, especially in risky places such as airports or hospitals.
Also, telemedicine is increasingly used to minimize risks as it aims to reduce hospital visits of groups such as the elderly, which are more vulnerable. Furthermore, telemedicine can also contribute to recovery ability and remote monitoring.
Healthcare monitoring systems include applications based on convolutional neural networks to detect a person falling, so-called human fall detection. Surveillance video can be used to detect the fall in combination with processing rules to automatically alert the caregivers.
Traffic and Infrastructure Monitoring
13. Smart City Traffic Monitoring
Computer Vision is used to analyze and predict traffic conditions. Real-time traffic data, including car count, frequency, and direction, can be generated using the video feed of common surveillance cameras. Vehicle counting uses deep neural networks to detect different vehicle types in high-traffic situations. Thus, using the information to optimize traffic management. Traffic monitoring also includes tracking of waiting times (dwell time tracking) and traffic flows.
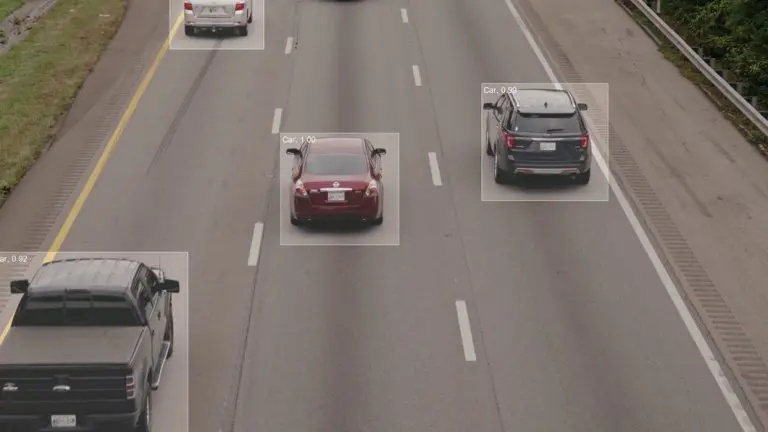
Detect and count different types of vehicles for traffic analysis.
14. Traffic Rule Violation Detection
Computer vision is used to analyze the huge amount of video surveillance data for traffic control in smart cities. This can aid in locating traffic rule violations. While traditional computer vision methods have been unable to analyze such a huge amount of visual data generated in real time, modern deep learning methods with Edge AI make it possible to find semantic patterns that are useful for interpretation.
An example is the automatic detection of bike riders without helmets in city traffic. Other use cases include the automatic recognition of situations that lead to road accidents to promote urban traffic safety and efficiency. Such applications include, for example, the detection of abnormal situations, such as vehicles that are stopping at dangerous locations.
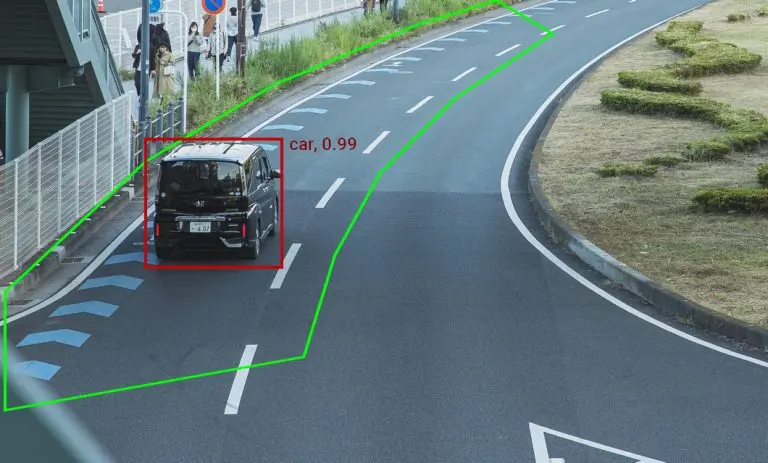
Detect unexpected vehicle stops, anomalies, and dangerous situations automatically.
15. Roadside Surveillance Application
Double parking and busy roadside activities such as frequent loading and unloading of trucks can also have a critical impact on traffic situations in cities with high transportation density. Here, computer vision applications with real-time surveillance of roadside loading and unloading bays are needed to detect roadside occupation automatically.
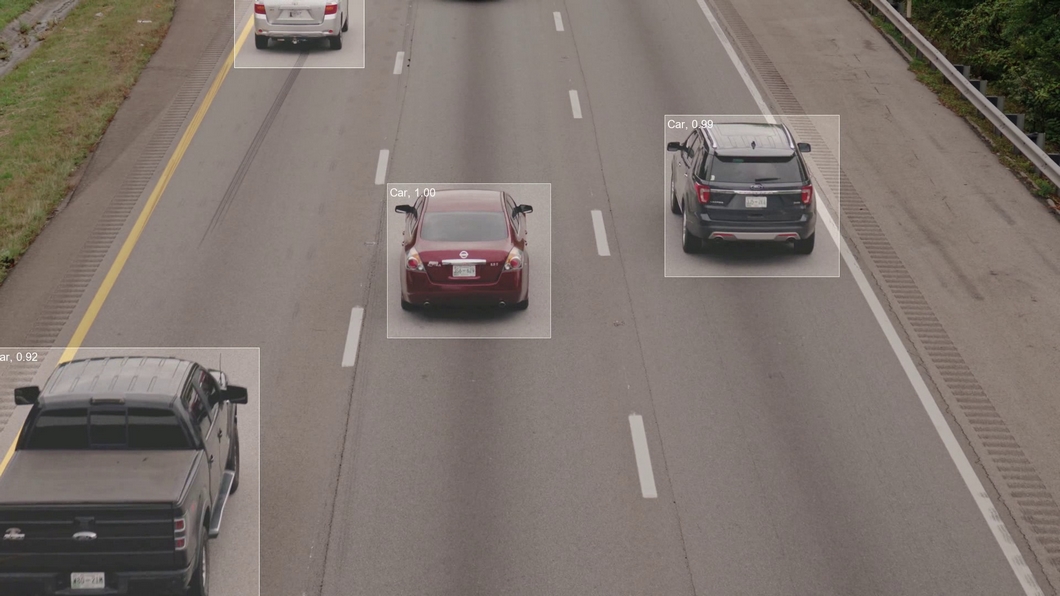
16. Parking Lot Occupancy Detection
Real-time parking lot occupancy detection recently gained a lot of importance. Solutions based on computer vision showed good performance in terms of accuracy and can be implemented using existing camera networks. Deep Learning is used to detect vacant parking lots over multiple cameras.
Novel Edge AI capabilities make it possible to analyze visuals close to the sensing devices without the need to transmit video streams to a central controller to acquire, encode, analyze, and process the images. Hence, distributed parking lot occupancy detection yields better performance also in terms of overall energy efficiency and accuracy.
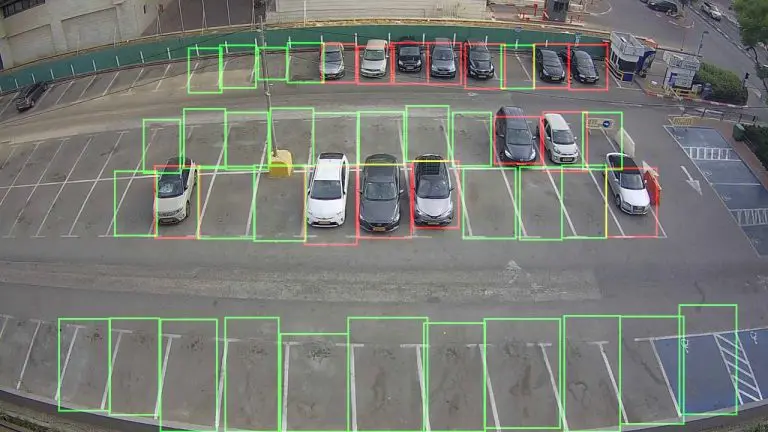
Complete application to detect vehicles with Computer Vision and Deep Learning
Other Smart City Computer Vision Applications
- Non-compliance detection at swimming pools, lakes, and rivers
- Detect littering, illegal waste disposal, and pollution
- Recognize incorrectly placed electric bikes, scooters, and other vehicles
- Trespassing on restricted footpaths or railway property
- Detection of abandoned vehicle removal and disposal
- Large-scale AI camera usage to assess road conditions
- Intelligent power management based on infrastructure usage
- Real-world data for intelligent utility management in cities
Get started with Computer Vision in Smart City
The opportunity for computer vision in smart city use cases is largely untapped. Novel technologies like robust Deep Learning models can be easily re-applied in a wide range of situations and capabilities. This makes computer vision scalable in real-time use cases have great potential to provide significant economic value to societies and cities.
Computer vision application platforms make it possible to develop such applications for smart cities. Therefore, camera streams are analyzed in real-time with AI models in combination with workflow logic to automate specific tasks (counting, warning, insights for decision-making).
Check out Viso Suite, the state-of-the-art computer vision infrastructure solution that offers scalable automated features to rapidly build and test such computer vision applications and run entire Edge AI systems on fully managed infrastructure.
Find more computer vision applications in related sectors: